CS methods applied to genomic research could effectively analyze large biobank dataset
10-15-2021
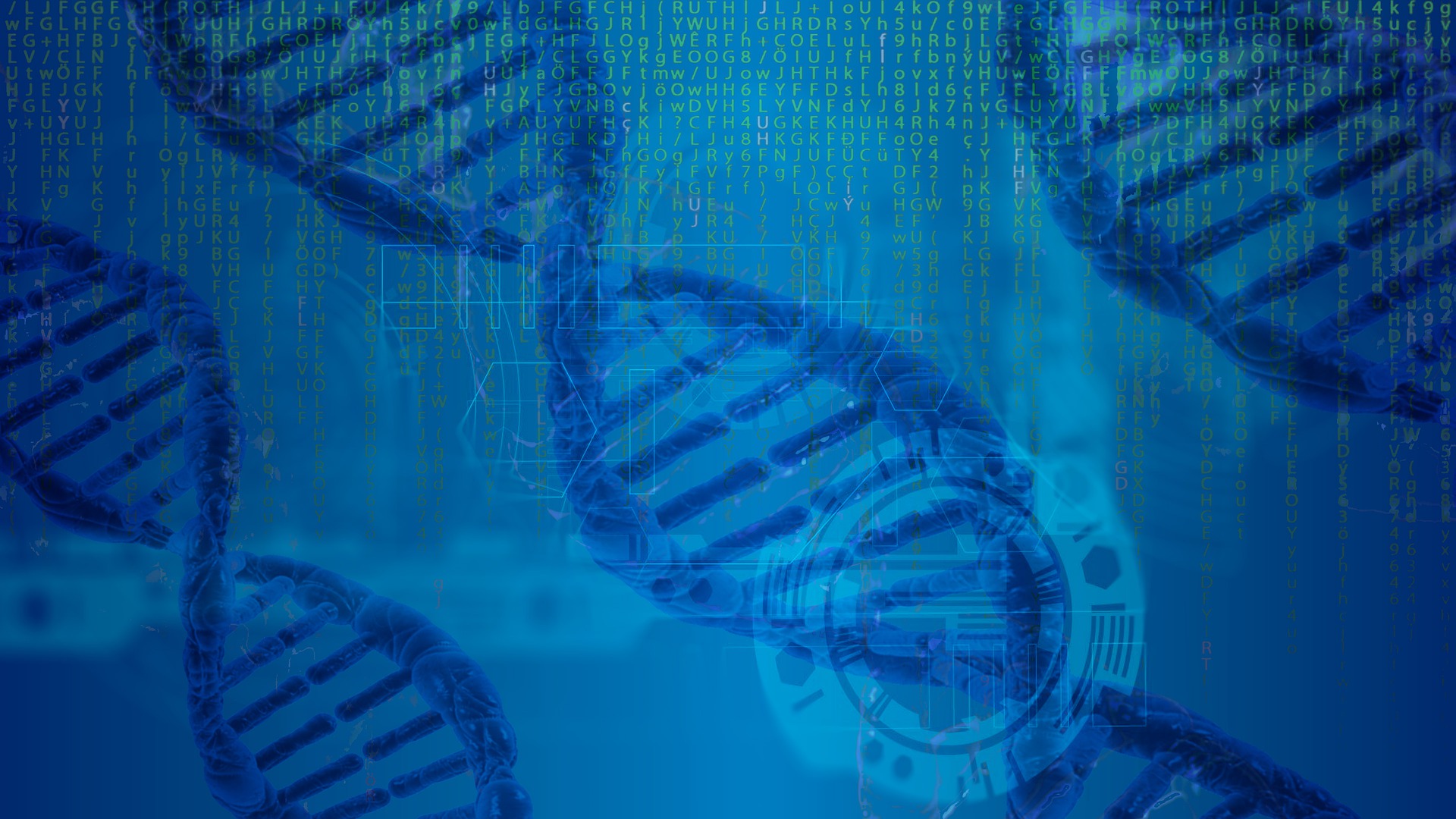
The analysis of human genetic data holds the promise of revolutionizing human health in the 21st century. Analyzing such datasets is how researchers can best find genes affecting health, disease, and responses to drugs and environmental factors, as well as understanding the evolutionary and biological history of our species.
The greatest challenge geneticists will soon face is the task of analyzing massive datasets with several millions of individuals and several tens of millions of genetic makers. Currently, the methods of analysis that have been applied in smaller studies have become computationally intractable.
To solve this problem, Purdue Computer Science Professor, Petros Drineas, suggests utilizing algorithms and methods from computer science and applied mathematics literature and then applying them to genomics research. With this multidisciplinary approach, we could see the development of novel tools that could unlock the potential to efficiently analyze biobank-scale data.
For his work in this area, Drineas has been awarded an IBM Academic Award for 2021. The award is a world-wide monetary program that promotes research and innovation between IBM and universities.
Large-scale genomic and phenotypic datasets exist from public cohorts of hundreds of thousands of individuals, such as the UK Biobank (UKB) project, NIH’s All of Us project, and the Million Veterans Program. These biobanks have already offered unprecedented opportunities to seek out biological pathways that underlie complex human traits and disease risk.
The aim of Drineas’ project is to use Linear Mixed Models (LMMs) to identify and quantify genetic risk, with great advantages for the study of data matrices on a biobank-scale.
“LMMs are emerging as a method of choice to conduct genetic association studies with benefits that include preventing false-positive associations due to population or relatedness structure and increased power,” said Drineas.
LMMs postulate a linear model for the genetic effects of the genotyped markers on the phenotype of interest. They begin by computing the Genetic Relatedness Matrix (GRM), which is a square, symmetric dense matrix, which for UKB-sized datasets has hundreds of thousands of rows and columns. After that, a maximum-likelihood estimator is used to compute the heritability parameters, followed by matrix inversion and matrix vector products in order compute the effect of each biological marker.
Most of these steps do not scale to biobank-scale as the underlying optimization problems are intractable. The work of Drineas’ project aims to speed up LMM computations without sacrificing accuracy for the analysis of biobank-scale data, by using matrix sketching algorithms, randomized linear algebra, and developing related software. The work will be done jointly with Dr. Laxmi Parida and Dr. Aritra Bose at IBM Research.
Petros Drineas is professor and associate head in the Department of Computer Science at Purdue University. He is known for his contributions to the theory of data science and the development of Randomized Numerical Linear Algebra (RandNLA). He received his PhD in Computer Science from Yale University in 2003. His research interests lie in the design and analysis of randomized algorithms for linear algebraic problems, as well as their applications to the analysis of modern, massive datasets. In a 2012 paper, he introduced CUR matrix approximation for improved big data analysis. Drineas' work on the application of principle component analysis to population genetics disproved the long-standing hypothesis that the Minoan civilization had North African origins.
About the Department of Computer Science at Purdue University
Founded in 1962, the Department of Computer Science was created to be an innovative base of knowledge in the emerging field of computing as the first degree-awarding program in the United States. The department continues to advance the computer science industry through research. US News & Reports ranks Purdue CS #20 and #18 overall in graduate and undergraduate programs respectively, ninth in both software engineering and cybersecurity, 14th in programming languages, 13th in computing systems, and 24th in artificial intelligence. Graduates of the program are able to solve complex and challenging problems in many fields. Our consistent success in an ever-changing landscape is reflected in the record undergraduate enrollment, increased faculty hiring, innovative research projects, and the creation of new academic programs. The increasing centrality of computer science in academic disciplines and society, and new research activities - centered around data science, artificial intelligence, programming languages, theoretical computer science, machine learning, and cybersecurity - are the future focus of the department. cs.purdue.edu
Writer: Emily Kinsell, emily@purdue.edu
Source: Petros Drineas, pdrineas@purdue.edu