CS Faculty Projects Among Winning Research Proposals in Integrative Data Science Initiative
05-23-2018
Writer(s): Kristyn Childres
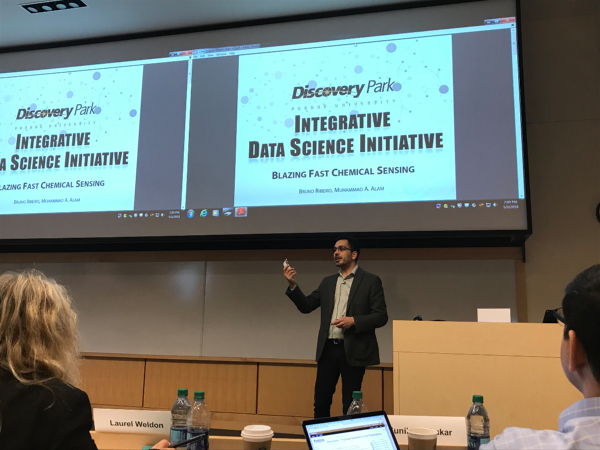
Computer science faculty members are involved in five of eight integrative data science research projects, launched this week as part of Purdue’s Integrative Data Science Initiative (IDSI). Announced by Purdue in April, the IDSI focuses on applying data science research to pressing fundamental issues while establishing an educational ecosystem of data fluency to prepare students for the rapidly expanding future of a data-driven, knowledge economy. Sunil Prabhakar, professor and head of the computer science department, has been appointed to lead this rapidly advancing initiative as the inaugural IDSI director.
The eight winning projects were selected from more than 50 applications that resulted from a call for ambitious proposals applying data science to pressing societal issues. These projects explore data science questions at the nexus of health care; defense; ethics, society and policy; and fundamentals, methods and algorithms.
“The involvement of computer science faculty in these projects underscores the centrality of computer science to data science,” said Prabhakar. “The group of selected projects will harness Purdue’s research power to tackle socially relevant issues.”
Below, read about projects from computer science faculty members.
Causally-Driven Healthcare Science – From Observational and Experimental Studies to Personalized and Improved Patient Outcomes
PI: Elias Bareinboim (Assistant Professor, Department of Computer Science)
Co-PIs: Pavlos Vlachos (Professor, Department of Mechanical Engineering), Mohammad Adibuzzaman (Research Scientist, Regenstrief Center for Healthcare Engineering)
In medicine, evidence generation relies on identifying the causal effectiveness of an intervention (clinical guideline, drug administration) based on randomized controlled trials (RCTs), which are expensive, time-consuming and sometimes unethical.
This project aims to develop new methods for causal identification for evidence generation in medicine. It will tackle the causal generalization problem in three broad specific applications in healthcare: i) identifying causality from experimental and observational data, ii) expediting randomized controlled trials with efficient design, and iii) methods for personalized medicine.
Quantum Machine Learning for Data Analytics and Optimization
PI: Sabre Kais (Professor, Department of Chemistry)
Co-PI: Alex Pothen (Professor and Associate Department Head, Department of Computer Science), Yong P. Chen (Professor, Department of Physics and School of Electrical and Computer Engineering), and Muhammad A. Alam (Jai N. Gupta Professor, School of Electrical and Computer Engineering)
Machine learning techniques are demonstrably powerful tools displaying remarkable success in compressing high dimensional data. These methods have been applied to a variety of fields in both science and engineering.
This project will build upon Purdue’s expertise in data science, machine learning and quantum computing by developing game-changing quantum algorithms to perform machine-learning tasks on large-scale scientific data sets for various industrial and technological applications based on optimization.
Formal Methods for Robust Machine Learning
PI: Jennifer Neville (Associate Professor and Miller Family Chair, Department of Computer Science)
Co-PIs: Dan Goldwasser, Bruno Ribeiro, Roopsha Samanta and Tiark Rompf (all Assistant Professors in the Department of Computer Science)
While there has been a great deal of success recently in the development of machine learning methods and systems for real world applications, much of the success has been in relatively restricted domains where there is (i) a clearly defined task, with (ii) clearly defined measures of success, (iii) large amounts of labeled training examples, (iv) limited structural variation, and (v) few system constraints. This proposal takes a two-pronged approach to develop more robust and efficient ML systems by using systems engineering principles and practices to improve the deployment of ML systems, and developing foundational methodology, analysis tools, and algorithms in machine learning to enable deployment in more realistic applications.
Blazing Fast Chemical Sensing
PI: Bruno Ribeiro (Assistant Professor, Department of Computer Science)
Co-PI: Muhammad A. Alam (Jai N. Gupta Professor, School of Electrical and Computer Engineering)
The field of chemical sensors is about to go through a seismic shift. Printed on top of biodegradable substrates, these revolutionary chemical sensors are designed to be short-lived, cost a few cents, and be ultra-low powered. Applications of these chemical sensors range from environmental monitoring, to precision agriculture, to health monitoring and beyond.
This project plans to develop hybrid data-driven and physics-driven techniques that can significantly speed up the time to get reliable sensor readings from unreliable electrical signals. If successful, this project will result in a tool that any lay practitioner can use to automatically build new mixed data-driven and physics-driven models that give reliable chemical concentration estimates in a matter of minutes, as opposed to hours.
A Relational-Based Measure of State Legislator Consequence
PI: Eric Waltenburg (Professor, Department of Political Science)
Co-PI: Dan Goldwasser (Assistant Professor, Department of Computer Science)
Understanding and accurately predicting legislative outcomes is essential to the development and pursuit of public policy. Ultimately, the passage of any public law is the result of the decisions of individual legislators. This project leverages Purdue’s strengths in computer science and unstructured data analytics to build a relational database of state legislative roll call votes and derive a consequence score for individual state legislators in all 50 states. It will draw on external, Internet-based data sources to test systematic explanations for the individual legislator consequence scores, and it will result in a database containing historical records of state legislators’ online activity annotated by policy frame, issue area, legislator stance or position, ideology, and other attributes.